Harnessing predictive analytics in orthopaedics: The power of Artificial Intelligence
By Amin Abukar
Trauma & Orthopaedic Registrar ST7, University College London Hospitals Rotation
With expanding applications of artificial intelligence (AI) and digital technology in orthopaedic surgery, there is growing awareness of the enhanced efficiency afforded by these tools. Substantial data generated in healthcare settings can be exploited using pattern recognition to highlight variations that allow for predictive accuracy. Insights afforded by AI have potential to provide value when navigating patient care pathways thereby aiding decision-making processes spanning preoperative considerations, identification of potential complications and outcomes including response to treatment. By enabling the provision of effective care plans, these models may also offer economic value by increasing operational efficiency. Spanning the last two decades, the number of studies focusing on predictive analytics in orthopaedic surgery have shown exponential growth (see Figure 1). There is mounting interest in predictive analytics within the realm of orthopaedic surgery however few models are utilised in clinical practice. Clinical applications of these models are still considered to be in their nascency, nonetheless studies in the literature continue to emphasise the efficiency of predictive algorithms.
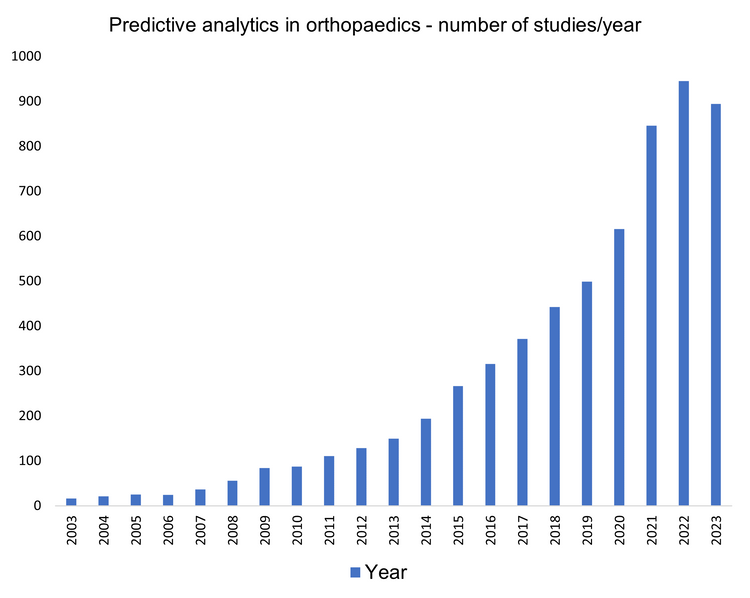
Figure 1: PubMed search for predictive analytics in orthopaedic surgery.
AI algorithms can show superiority in predicting risks relating to morbidity and mortality relative to scoring systems conventionally utilised in clinical settings. These algorithms have been exploited in the field of spinal surgery, in particular adult spinal deformity (ASD). A study conducted by Kim et al.1 employed AI to anticipate postoperative complication rates amongst adult patients undergoing surgery to correct spinal deformities. The team utilised data to train and validate machine learning models to identify factors which may pose risks for complications following surgery. Selection of predictive variables prove key to these models, some variables utilised in this study comprise sex, age, diabetes, ethnicity, specific comorbidities, American Society of Anaesthesiologists (ASA) class >3 etc. ASA class provided a reference point for predictions with accuracy of models validated used area under operating characteristic curves (AUC), see Figure 2. AI subfields such as logistic regression (LR) and artificial neural networks (ANNs) were able to predict risks including cardiac complications, wound complications, venous thromboembolism (VTE) as well as mortality. Highlighting their value in risk factor analysis, machine learning models outperformed ASA scoring in the prediction of individual risk prognosis. Notably ANN demonstrated superior performance relative to LR in predicting certain risks (cardiac complications, wound complications as well as mortality) where (p < 0.05). As these tools utilise training algorithms on clinical data, a notable advantage is ongoing learning; these tools can enhance their predictive algorithms by continuously learning from data such that the performance of algorithms enhances over time. These models present as effective additions to decision making processes in the surgical setting however further studies are required to demonstrate clinical utility1.
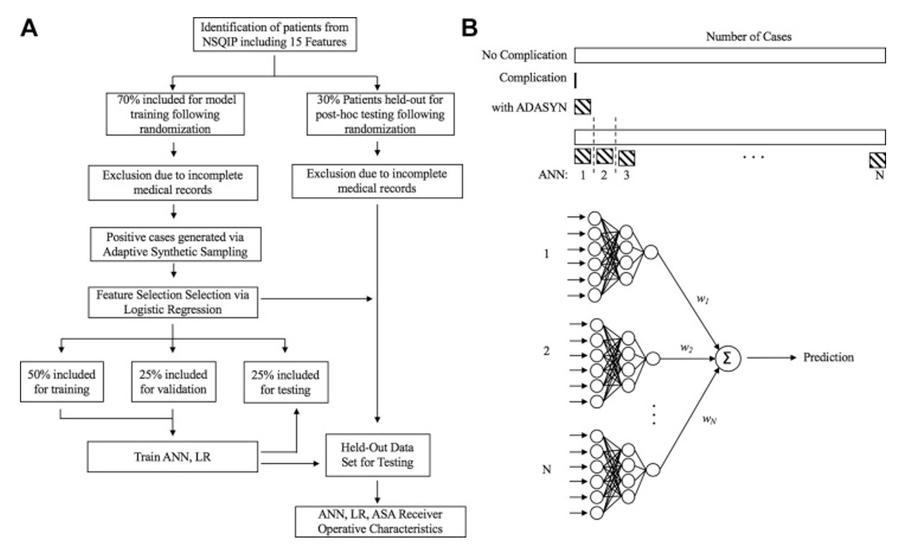
Figure 2: A. Patient selection and use of data to train and evaluate models. B. Predictive output1.
AI tools can also support clinical decisions in primary care for low back pain, with 90% of cases regarded as non-specific. Predictive analytics can therefore be used to provide recommendations which guide decision making. Analytics were used to identify patient subgroups, with the primary study purpose listed as identifying subgroups with modifiable risk factors, addressing these risk factors could therefore support treatment outcomes. For example, in the management of low back pain, physiotherapy referrals are somewhat inconsistent, predictive tools could therefore allow for a more systematic approach by ensuring key modifiable indicators are not overlooked. In the screening tool, allocation to the ‘high risk’ subgroup was motivated by psychosocial prognostic indicators such as fear, anxiety, depression, therefore patients were allocated to the high-risk subgroup provided overall tool score was greater than or equal to ≥4. Individuals rated ‘high risk' would benefit from counselling, where measures like this would be detrimental in those with conservative pain, this tool may therefore help guide early secondary prevention in primary care settings2.
Furthermore, machine learning models can help identify patients where recovery may be compromised by prolonged use of analgesia secondary to arthroscopy. With their ease of implementation, these predictive analytics could allow for prompt recognition of patients at risk, healthcare staff are therefore able to optimise modifiable risk factors therefore allowing for preoperative guidance/advice for patients, moving towards a patient-centred approach. Ease of deploying the current machine-learning model in clinical setting presents as a clear advantage, as the web-based application exploited in this study can be seamlessly integrated into patient visits thereby proving minimally disruptive in clinic. Providers can therefore identify and effectively manage patients deemed high risk. This study also shows that innovative approach of machine learning has broader applications in orthopaedic sports medicine. Applications can assist with risk stratification, outcome predictive, planning for postoperative pain management and prediction of clinically significant outcomes. Nonetheless, further research is required to determine whether patient outcomes are improved with utilisation of this tool. When considering limitations, it is not feasible that all potential variables that affect analgesic dependence secondary to hip arthroscopy have been include as prolonged opioid consumption remains a somewhat multifactorial phenomenon. Furthermore, with machine learning validation, the quality of the training data may be improved with accurate sample size determination which is also investigate at present. Prior to uptake in a clinical setting the appropriate external validation is crucial. Once again highlighting limitations despite overwhelming evidence in the literature, factors such as preoperative opioid use wasn’t identified by the tool as a significant risk factor which is most likely due to small numbers of positive cases in the training cohort which may have had minimal impact on model performance, therefore continuous learning and validation with data collected in a prospective manner would allow for a more accurate capture of the influence of key variables3.
Further applications of these tools that have been highlighted in the review by Khoriati and colleagues, these include the use of machine learning in to anticipate variances in patient reported outcomes subsequent to osteochondral graft transplantation (OCA) in knee surgery, predictive analysis in progression of knee arthritis as well as the potential requirement for hospital admission secondary to ACL surgery4.
Predictive analytics can be integrated into routine clinical practice through utilisation of decision support tools, growing literature highlights that the analysis of patient data and historical records can allow AI algorithms to support pre-emptive intervention offering a more personalised, systematic approach to patient care. Though research has highlighted the potential of predictive models to inform decision making, the success of predictive analytics requires significant amounts of high-quality data as sufficient predictive value will require inclusion of relevant data. Furthermore the tool utilised should be externally validated with establishment of a framework for ongoing monitoring and updates. Fortunately, continuous learning will allow AI models to showcase increased accuracy and reliability, with their ability to refine algorithms, AI models have the capacity self-assess performance thereby adjusting internal algorithms to enhance efficiency. This also highlights the importance of increased exposure to training datasets to optimise predictive analytics. Within orthopaedic surgery further studies are required to validate or counter predictive models whilst taking existing guidelines into consideration. With more research, predictive analysis models can be applied in clinic and theatres as digital applications that support real time decision making to optimise patient outcome.
References
- Kim JS, Arvind V, Oermann EK, Kaji D, Ranson W, Ukogu C, et al. Predicting Surgical Complications in Patients Undergoing Elective Adult Spinal Deformity Procedures Using Machine Learning. Spine Deform. 2018;6(6):762-70.
- Hill JC, Dunn KM, Lewis M, Mullis R, Main CJ, Foster NE, et al. A primary care back pain screening tool: Identifying patient subgroups for initial treatment. Arthritis Care Res (Hoboken). 2008;59(5):632-41.
- Lu Y, Forlenza E, Wilbur RR, Lavoie-Gagne O, Fu MC, Yanke AB, et al. Machine-learning model successfully predicts patients at risk for prolonged postoperative opioid use following elective knee arthroscopy. Knee Surg Sports Traumatol Arthrosc. 2022;30(3):762-72.
- Khoriati AA, Shahid Z, Fok M, Frank RM, Voss A, D’Hooghe P, et al. Artificial intelligence and the orthopaedic surgeon: A review of the literature and potential applications for future practice: Current concepts. J ISAKOS. 2024;9(2):227-33.