Integrating artificial intelligence into trauma and orthopaedics: History, current state of AI in T&O and future perspectives
By Andrew Coppolaa, Caroline Hingb, and Vipin Asopaa,b
a South West London Elective Orthopaedic Centre, Epsom and St. Helier University Hospitals NHS Trust
b St George's University Hospital, London.
Introduction
Artificial Intelligence (AI) aims to develop systems capable of executing tasks traditionally associated with human intelligence. Since its inception in the mid-20th century, AI has undergone significant evolution, transitioning from basic algorithms to complex artificial neural networks. The Dartmouth Conference of 1956 is widely recognised as a seminal event that marked the beginning of organised research efforts in AI1. Recent advancements in AI have been accelerated by innovations in cloud computing, which provide scalable computational resources, and improvements in processor technology, enhancing the speed and efficiency of complex algorithms and real-time analysis2,3.
In the field of orthopaedics, AI demonstrates significant potential by leveraging extensive visual data, including X-rays, MRI, and CT scans, alongside registry data from repositories such as the National Joint Registry and the American Joint Replacement Registry. This strategic integration of AI presents a significant opportunity to transform orthopaedic practice through enhanced diagnostic accuracy, refined surgical planning processes, and overall improvements in patient outcomes4-6. Moreover, AI facilitates the development of patient specific predictive models, representing a paradigm shift toward personalised medicine7,8. These insights underscore AI's emergent role as a transformative tool set to reshape orthopaedic care.
The purpose of this article is to discuss the history of AI, orthopaedic applications and future perspectives. The review will explore the implications of utilising extensive data sets, regulatory challenges, and the necessity for dynamic data solutions and continuous learning within the field.
History of artificial intelligence
The conceptual foundation of AI began in 1943 with McCulloch and Pitts introducing the idea of artificial neurons, inspired by earlier theoretical work by Alan Turing9,10. The field was formally established in 1956 at the Dartmouth Conference, recognised as the starting point for organised AI research1. Throughout the late 1950s, significant advancements such as the development of the ADALINE and MADALINE neural networks by Widrow and Hoff in 1959 laid the groundwork for later innovations in machine learning11. The introduction of the Von Neumann architecture in the 1960s dominated computing approaches and temporarily overshadowed neural network research12.
The 1970s marked the first 'AI winter', a period of reduced funding and interest due to unrealistically high expectations and subsequent disillusionment. Despite this, research continued, leading to key developments like the backpropagation technique in 1975, which improved the training efficiency of multi-layer neural networks13. AI experienced a resurgence in the 1980s with significant research contributions and commercial interest, rejuvenating the field. This period saw the development of cooperative-competitive neural networks and the popularisation of LISP machines, although another AI winter occurred late in the decade due to market saturation and a shift back to cheaper personal computing options14.
The 1990s ushered in a new era of practical AI applications, highlighted by IBM's Deep Blue defeating world chess champion Garry Kasparov in 199715. The following decade saw rapid advancements in GPU technology, enhancing the training of neural networks, and the introduction of user-interactive AI applications like Microsoft's Kinect and Apple’s Siri, broadening the public’s interaction with AI technologies. The 2010s were marked by breakthroughs in deep learning, notably through Geoffrey Hinton's work, which significantly advanced image and text analysis capabilities. His contribution to the development of convolutional neural networks culminated in winning the prestigious Turing award in 2018 (figure 1)16,17.
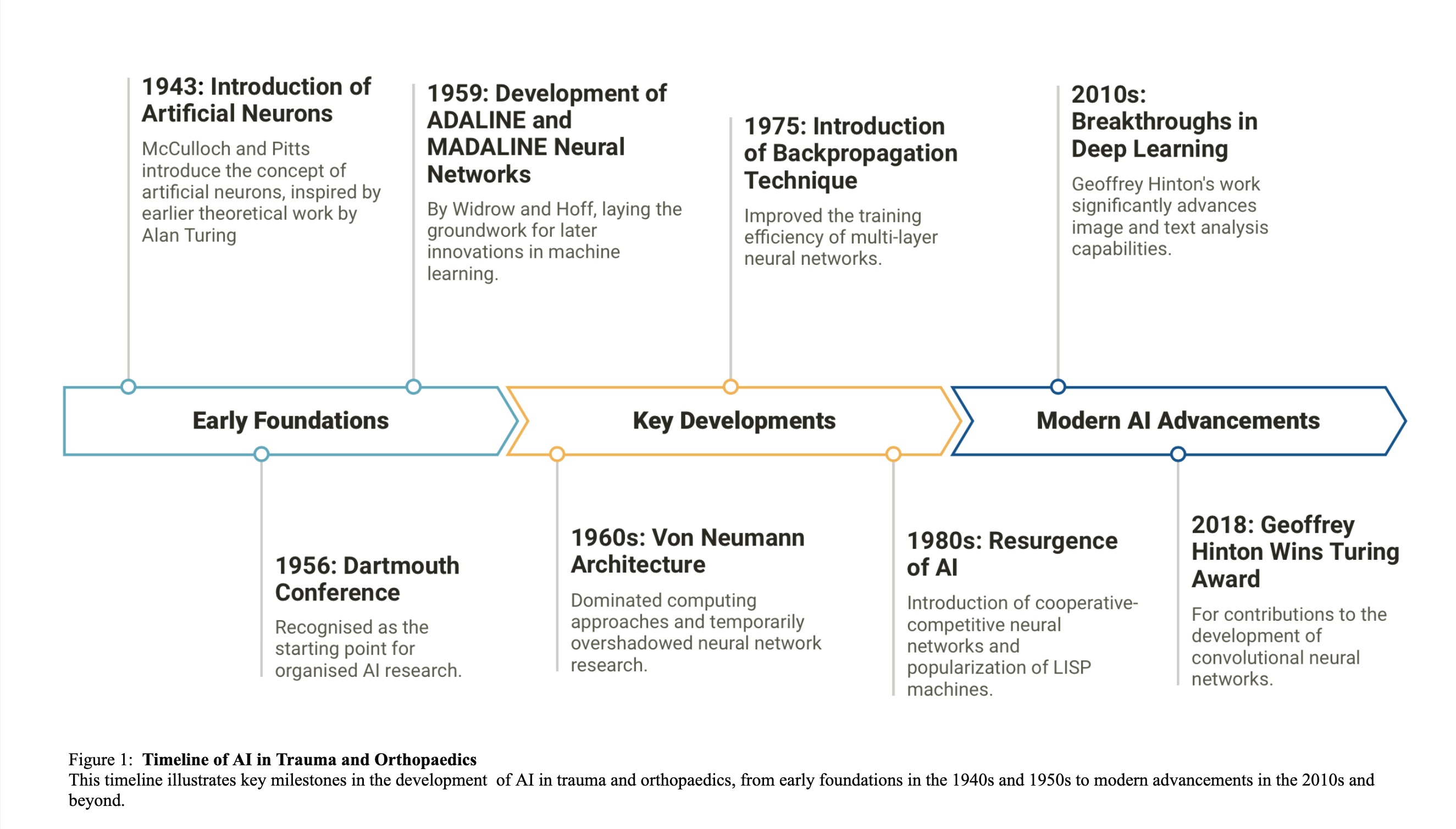
Today, AI is a diverse field that spans numerical analysis, text analysis through large language models, and image analysis, with applications affecting nearly every sector of society.
Current state of AI in trauma and orthopaedics
Recent advancements in AI in the field of trauma and orthopaedics have been propelled by breakthroughs in cloud computing and enhanced processor capabilities. These innovations enable scalable, virtual computing resources that significantly improve the processing speed and efficiency of complex algorithms, facilitating near real-time analysis of data. Although current clinical applications of AI in orthopaedics are limited, the extensive and diverse data available from orthopaedic settings — such as imaging and registries — hold the potential for significant developments.
Currently, AI applications in orthopaedics can be categorised into three main areas (figure 2):
- Predictive analytics: Utilising machine learning to forecast patient outcomes and optimise treatment plans.
- Computer vision: Enhancing diagnostics and surgical planning through advanced image analysis.
- Natural language processing (NLP): Streamlining documentation and extracting meaningful insights from clinical texts.
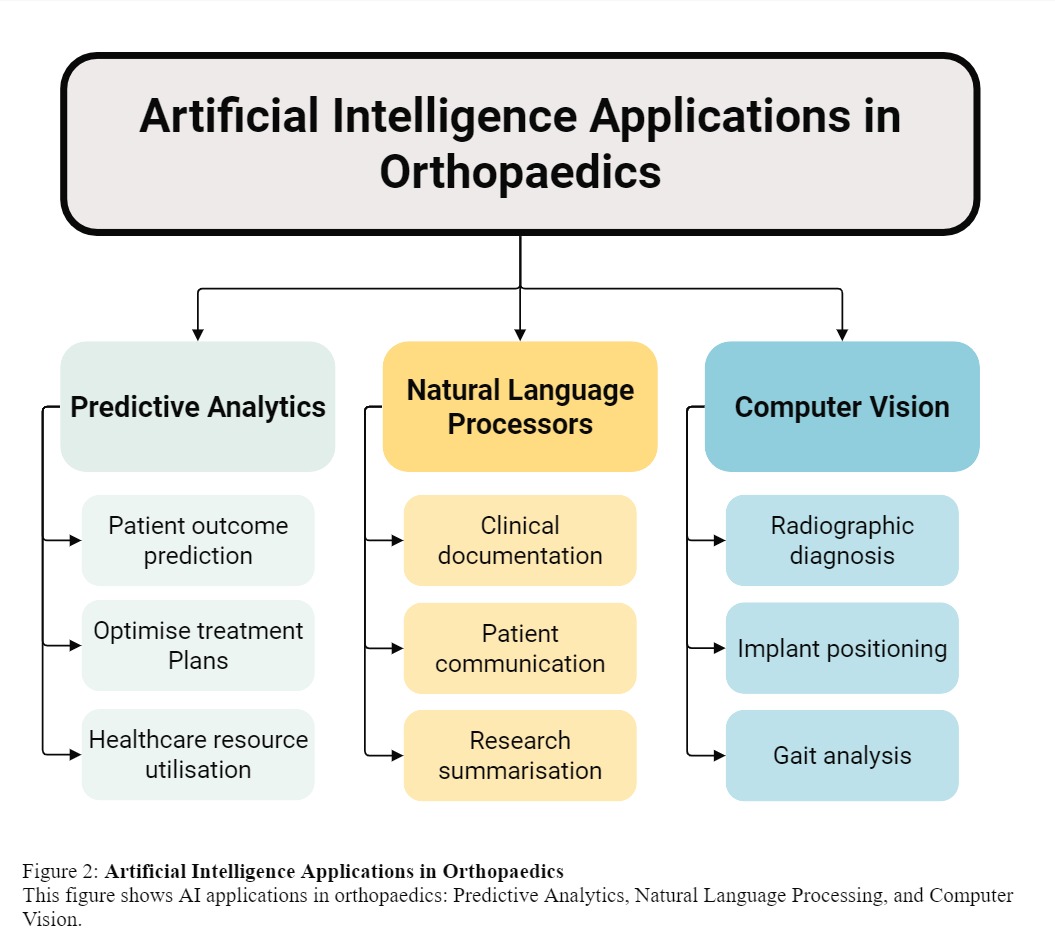
Predictive analytics
Predictive analytics harnesses machine learning and deep learning technologies to enable computers to learn from vast amounts of data and make accurate predictions. Machine learning focuses on identifying patterns and relationships in data, while its subset, deep learning, utilises complex neural networks that mirror the human brain's architecture to analyse data at an even deeper level18. These technologies have been transformative, especially in predicting patient outcomes such as treatment effectiveness, patient satisfaction, potential postoperative complications, duration of hospital stays, and the likelihood of readmission19-25.
In the field of orthopaedics, predictive analytics has proven particularly effective. Machine learning models analyse detailed surgical procedure information and patient-specific data, capturing complex and nonlinear relationships that traditional analytical methods may misinterpret26. This nuanced understanding allows healthcare providers to tailor care protocols more precisely, thus enhancing the quality of outcomes and overall patient experiences.
A study by Huber et al. effectively predicted patient-reported outcomes from hip and knee replacement surgeries by analysing over 130,000 observations from the NHS PROMs dataset27. The research utilised machine learning models, including techniques like extreme gradient boosting and random forests, which demonstrated high predictive accuracy with AUC scores of 0.87 and 0.86. Similarly, research by Chen et al. using over 246,000 patient records from the American College of Surgeons National Surgical Quality Improvement Program, showed AI's efficacy in predicting the length of hospital stay (LOS) for Total Hip Arthroplasty patients19. Further, a review by Spence et al. indicates that deep learning methods are highly accurate in predicting surgical case durations, which is crucial for optimising hospital workflows, resource allocation, and reducing surgical backlogs28. These advancements in AI-driven predictive analytics are poised to significantly improve efficiency, patient care, and hospital management in orthopaedics.
As predictive analytics continues to evolve, its use in orthopaedic research and practice is expected to become more widespread, leading to increasingly data-centric and personalised approaches to patient care. This evolution marks a significant shift towards leveraging data-driven insights to improve all aspects of orthopaedic care, from pre-surgical planning to post-operative recovery and follow-up.
Computer vision
Computer vision allows computers to 'see' and interpret images and videos, much like human vision. This area of AI relies on technologies such as Deep Convolutional Neural Networks (DCNNs), which mimic the way humans process visual information, and transformer models, advanced algorithms that understand the context and relationships within visual data29.
In orthopaedics, the application of computer vision has potential to revolutionise the analysis of medical imaging data, such as X-rays, MRI, and CT scans. This technology enables a computer to diagnose various conditions, detect fractures, identify soft tissue injuries, and classify injuries in accordance with established medical standards30. Moreover, it can evaluate the positioning and alignment of arthroplasty implants in postoperative images31.
The study by Yoon et al. assesses the application of DCNNs in the detection of scaphoid fractures, analysing a dataset of 11,838 radiographs32. The research demonstrated the DCNN's effectiveness in enhancing diagnostic accuracy for both apparent and occult fractures, with the model achieving a sensitivity of 87.1%, specificity of 92.1%, and an area under the receiver operating curve (AUROC) of 0.955. These findings indicate the capability of DCNNs to significantly improve the detection of scaphoid fractures, including those that might not be identified through conventional examination methods.
Advancements in DCNNs have demonstrated potential in improving the diagnosis of meniscal tears. A recent meta-analysis indicates that AI models are capable of accurately detecting the presence of meniscal tears when analysing MRI images, with reported pooled sensitivity and specificity rates suggesting favourable diagnostic performance33. These outcomes suggest that AI could play a significant role in supporting the diagnostic capabilities of clinicians and radiologists. The adoption of AI for the diagnosis of meniscal tears suggests a shift towards more accurate, data-driven, and efficient approaches in orthopaedic care. Nevertheless, the full realisation of AI's potential in this area is contingent upon addressing existing challenges, including the need for standardised reporting, external validation, and comprehensive analyses of AI's diagnostic methodologies.
Natural language processing
NLP is a branch of AI that enables machines to understand, interpret, and respond to human language in a way that is both valuable and meaningful34. Patients are increasingly utilising technologies like ChatGPT for summarising information regarding healthcare issues, indicating a growing reliance on AI for health-related guidance. Despite its utility, there are concerns regarding the accuracy of the information provided and the potential for 'hallucinations', a term in AI that refers to instances where the model generates false or misleading information not supported by the input data. These challenges underscore the need for careful evaluation of AI-generated health information to ensure its reliability and safety for patient use.
In a study of 68 orthopaedic experts, ChatGPT's advice on post-surgical self-management was assessed for accuracy, applicability, and comprehensiveness35. Although it scored highly in accuracy and applicability, it was found lacking in depth, highlighting opportunities for enhancement to better address patient informational needs. Furthermore, NLP has potential to transform orthopaedic research by converting unstructured data — such as clinical notes, patient-reported narratives, and imaging reports — into structured formats, thereby facilitating the exploration of new research avenues. NLP can further support practitioners by efficiently summarising the latest research and treatment updates, and aid in clinical decision-making by extracting critical information from various health record sources, facilitating more informed and streamlined treatment planning.
While NLP holds promise for revolutionising orthopaedic medicine by streamlining tasks, enhancing information access, and supporting research efforts, it also faces limitations. These include potential inaccuracies in data interpretation, the need for extensive training datasets that accurately reflect clinical scenarios, and ensuring that the technology's decisions are both understandable and trusted by users
Current challenges and future perspectives
Implementing AI in orthopaedic surgery offers a significant opportunity to transform the practice, enhancing diagnostic accuracy, refining surgical planning, and potentially enabling semi- or fully autonomous procedures to improve outcomes. Beyond surgery, AI can streamline the entire care process — from primary care referrals to efficient clinic bookings and communications. Automated data gathering and reporting can revolutionise clinic assessments, real-time recording and transcription, appointment scheduling, and the allocation of operating slots and resources. Additionally, AI can tailor patient consents, rehabilitation protocols, and follow-up appointments to individual needs, boosting NHS efficiency and reducing costs.
Realising these benefits requires overcoming substantial challenges. Regulatory, ethical, and methodological hurdles, such as ensuring privacy, enhancing the consent process, and addressing the 'right to be forgotten' in data use, pose significant obstacles. Further, AI's 'black box' nature necessitates greater transparency and accountability to ensure that both healthcare providers and patients understand how decisions are made36. Addressing potential biases in AI systems is crucial to prevent care disparities, requiring diverse and representative training datasets. Initiatives like TRIPOD-AI and PROBAST-AI are crucial in establishing standards to ensure reliability and transparency in AI development37,38.
As we look to the future, interdisciplinary collaboration among computer scientists, data analysts, orthopaedic surgeons, and other healthcare professionals will be vital for advancing AI technologies within clinical settings. A key aspect of this advancement is the development of dynamic AI systems that not only adapt but also continuously learn from ongoing clinical data. The concept of 'data half-life' — the period after which data may become less relevant — underscores the need for AI models that can update their knowledge base in real-time to reflect the latest medical insights39. An adaptive methodology that incorporates various models and promotes continuous learning is crucial for enhancing the efficacy and reliability of AI applications. By embracing these dynamic models, we can significantly improve the flexibility and clinical utility of AI systems, paving the way for truly personalised medicine. This approach is a promising direction that aims to transform patient care by tailoring treatments to individual needs based on the most current and relevant data40.
References
- McCarthy J, Minsky ML, Rochester N, et al. A proposal for the Dartmouth summer research project on artificial intelligence. AI Mag 2006;27:12-4.
- Lins S, Pandl KD, Teigeler H, et al. Artificial Intelligence as a Service - Classification and Research Directions. Bus Inf Syst Eng 2021;63(4):441-56.
- Taivalsaari A, Mikkonen T. A Roadmap to the Programmable World: Software Challenges in the IoT Era. IEEE Softw 2017;34:72-80.
- Hendrix N, Scholten E, Vernhout B, et al. Development and validation of a convolutional neural network for automated detection of scaphoid fractures on conventional radiographs. Radiol Artif Intell 2021;3:4.
- 5Kunze KN, Polce EM, Patel A, et al. Machine learning algorithms predict within one size of the final implant ultimately used in total knee arthroplasty with good-to-excellent accuracy. Knee Surg Sports Traumatol Arthrosc 2022;30:2565-72.
- Lisacek-Kiosoglous AB, Powling AS, Fontalis A, et al. Artificial intelligence in orthopaedic surgery. Bone Joint Res 2023;12(7):447-54.
- Yoo HJ, Jeong HW, Kim SW, et al. Prediction of progression rate and fate of osteoarthritis: Comparison of machine learning algorithms. Journal of Orthopaedic Research 2023;41:583-90.
- Katakam A, Karhade A V, Collins A, et al. Development of machine learning algorithms to predict achievement of minimal clinically important difference for the KOOS-PS following total knee arthroplasty. J Orthop Res 2022;40(4):808-15.
- Turing A. Computing Machinery and Intelligence. Mind 1950;49:433-60.
- McCulloch WS, Pitts W. A logical calculus of the ideas immanent in nervous activity. Bull Math Biophys 1943;5:115-33.
- Widrow, B. and Hoff, M. E. (1960). Adaptive switching circuits.
- Von Neumann J, Godfrey MD. First Draft of a Report on the EDVAC. IEEE Annals of the History of Computing 1993;15(4):27-75.
- Rumelhart DE, Hinton GE, Williams RJ. Learning representations by back-propagating errors. Nature 1986;323:533-6.
- Schuchmann S. History of the Second AI Winter. Towards Data Science (2019). Available at: https://towardsdatascience.com/history-of-the-second-ai-winter-406f18789d45.
- Hsu F-H. Behind Deep Blue: Building the computer that defeated the world chess champion. Princeton University Press, 2002.
- Krizhevsky A, Sutskever I, Hinton GE. ImageNet classification with deep convolutional neural networks. Commun ACM 2017;60:84–90.
- Schmidhuber J. Scientific Integrity and the History of Deep Learning: The 2021 Turing Lecture, and the 2018 Turing Award. Technical Report IDSIA-77-21 (v3), IDSIA, Lugano, Switzerland, 2021–2022, 2022.
- Kriegeskorte N, Golan T. Neural network models and deep learning. Current Biology 2019;29:R231-R236.
- Chen TLW, Buddhiraju A, Costales TG, et al. Machine Learning Models Based on a National-Scale Cohort Identify Patients at High Risk for Prolonged Lengths of Stay Following Primary Total Hip Arthroplasty. J Arthroplasty 2023;38:1967-72.
- Lin-Wei Chen T, Buddhiraju A, Hojoon Seo H, et al. Can machine learning models predict prolonged length of hospital stay following primary total knee arthroplasty based on a national patient cohort data? Arch Orthop Trauma Surg 2023;143(12):7185-93.
- Kim JS, Merrill RK, Arvind V, et al. Examining the Ability of Artificial Neural Networks Machine Learning Models to Accurately Predict Complications Following Posterior Lumbar Spine Fusion. Spine (Phila Pa 1976) 2018;43:853-60.
- Harris AHS, Kuo AC, Weng Y, et al. Clinical Research Can Machine Learning Methods Produce Accurate and Easy-to-use Prediction Models of 30-day Complications and Mortality After Knee or Hip Arthroplasty? Clin Orthop Relat Res 2019;477:452-60.
- Garriga C, Sanchez-Santos MT, Judge A, et al. Development of a model predicting non-satisfaction 1 year after primary total knee replacement in the UK and transportation to Switzerland. Sci Rep 2018;8(1):3380.
- Nam HS, Ho JPY, Park SY, et al. The development of machine learning algorithms that can predict patients satisfaction using baseline characteristics, and preoperative and operative factors of total knee arthroplasty. Knee 2023;44:253-61.
- Park J, Zhong X, Miley EN, et al. Machine Learning-Based Predictive Models for 90-Day Readmission of Total Joint Arthroplasty Using Comprehensive Electronic Health Records and Patient-Reported Outcome Measures. Arthroplast Today 2023;25:101308.
- Bertsimas D, Dunn J, Velmahos GC, et al. Surgical Risk Is Not Linear: Derivation and Validation of a Novel, User-friendly, and Machine-learning-based Predictive OpTimal Trees in Emergency Surgery Risk (POTTER) Calculator. Ann Surg 2018;268:574-83.
- Huber M, Kurz C, Leidl R. Predicting patient-reported outcomes following hip and knee replacement surgery using supervised machine learning. BMC Medical Informatics and Decision Making. 2019;19:1-13.
- Spence C, Shah OA, Cebula A, et al. Machine learning models to predict surgical case duration compared to current industry standards: scoping review. BJS Open 2023;7:zrad113.
- Szeliski R. Computer vision: algorithms and applications.
- Yang S, Yin B, Cao W, et al. Diagnostic accuracy of deep learning in orthopaedic fractures: a systematic review and meta-analysis. Clin Radiol 2020;75:713.e17-713.e28.
- Shah AK, Lavu MS, Hecht CJ, et al. Understanding the use of artificial intelligence for implant analysis in total joint arthroplasty: a systematic review. Arthroplasty 2023;5:1-12.
- Yoon AP, Lee YL, Kane RL, et al. Development and Validation of a Deep Learning Model Using Convolutional Neural Networks to Identify Scaphoid Fractures in Radiographs. JAMA Netw Open 2021;4:e216096–e216096.
- Zhao Y, Coppola A, Karamchandani U, et al. Artificial intelligence applied to magnetic resonance imaging reliably detects the presence, but not the location, of meniscus tears: a systematic review and meta-analysis. Eur Radiol. Epub ahead of print 2024. DOI: 10.1007/s00330-024-10625-7.
- Deng L, Yu D. Deep learning: Methods and applications. Foundations and Trends in Signal Processing; 7. Epub ahead of print 2013. DOI: 10.1561/2000000039.
- Yapar D, Avcı YD, Sonuvar ET, et al. ChatGPT’s potential to support home care for patients in the early period after orthopedic interventions and enhance public health. Jt Dis Relat Surg; 35. Epub ahead of print 2023. DOI: 10.52312/JDRS.2023.1402.
- Durán JM, Jongsma KR. Who is afraid of black box algorithms? On the epistemological and ethical basis of trust in medical AI. J Med Ethics 2021;47:329-35.
- Wolff RF, Moons KGM, Riley RD, et al. PROBAST: A tool to assess the risk of bias and applicability of prediction model studies. Ann Intern Med 2019;170:51-8.
- Heus P, Damen JAAG, Pajouheshnia R, et al. Poor reporting of multivariable prediction model studies: Towards a targeted implementation strategy of the TRIPOD statement. BMC Med 2018;16:1–12.
- Chen JH, Alagappan M, Goldstein MK, et al. Decaying relevance of clinical data towards future decisions in data-driven inpatient clinical order sets. Int J Med Inform 2017;102:71-9.
- Hadsell R, Rao D, Rusu AA, et al. Embracing Change: Continual Learning in Deep Neural Networks. Trends Cogn Sci 2020;24:1028-40.